|
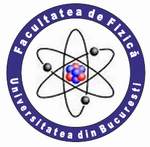 |
UNIVERSITY OF BUCHAREST FACULTY OF PHYSICS Guest 2025-07-01 12:47 |
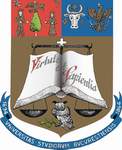 |
|
|
Conference: Bucharest University Faculty of Physics 2011 Meeting
Section: Biophysics; Medical Physics
Title: Compressed Sensing in Magnetic Resonance Imaging
Authors: Radu Mutihac
Affiliation: Department of Physics, University of Bucharest, ROMANIA.
Division of Psychiatry and Neuroscience, WRAIR, Silver Spring, MD 20910, USA.
Division of Intramural Research, NIDCD/NIH, Bethesda, MD 20892, USA.
E-mail radu.mutihac.ctr@us.army.mil
Keywords: compressed sensing, magnetic resonance imaging, image reconstruction, sampling, k-space, wavelets, sparsifying transform.
Abstract: The sampling theorem is a fundamental result of information theory having direct impact on signal processing. Perfect reconstruction is proven mathematically possible for idealized models as the theorem applies to signals that are sampled for infinite time only. The sampling theorem provides a sufficient condition for perfect reconstruction, though not a necessary one.
Compressed sensing (CS) yields a stricter sampling condition when underlying signals are sparse and it demonstrates a sub-Nyquist sampling criterion.
Most natural images can be compressed with minimal perceptible loss of information. Transform-based compression is adopted in standards like JPEG or MPEG. Essentially, the strategy consists in applying a sparsifying transform that maps image content into a vector of sparse coefficients, and subsequently encoding the sparse vector by approximation of the most significant coefficients and discarding the smaller ones.
Application of CS is conditioned by:
- Transform sparsity: sparsely represented image in a transform domain (wavelets);
- Incoherence of undersampling artifacts: incoherent (noise like) artifacts in the sparsifying transform domain caused by k-space undersampling in linear reconstruction;
- Nonlinear reconstruction: reconstructed images by a nonlinear method that enforces both sparsity of the image representation and consistency of the reconstruction with the acquired samples.
Magnetic resonance imaging (MRI) has emerged as a non-invasive medical imaging technique, yet limited by slow data acquisition. Any CS protocol for MRI amounts to selecting a subset of frequency domain that can be efficiently sampled and is incoherent with respect to the sparsifying transform. As such, CS is able to make accurate reconstructions from a small subset of k-space, rather than an entire k-space grid. Applying CS to MRI entails significant scan time reductions that are beneficial for large data processing and effective diagnosis.
The research has been supported by the NAS/NRC Contract W81XWH-07-2-0001-0114.
Donoho D. L. (2006), “Compressed sensing.” IEEE Trans Inf Theory, 52(4): 1289-306.
|
|
|
|