|
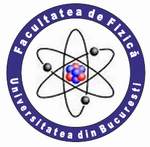 |
UNIVERSITY OF BUCHAREST FACULTY OF PHYSICS Guest 2025-04-04 21:35 |
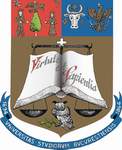 |
|
|
Conference: Bucharest University Faculty of Physics 2006 Meeting
Section: Electricity and Biophysics
Title: Exploratory Analysis of Functional Brain Imaging Data
Authors: Radu Mutihac
Affiliation: Department of Electricity and Biophysics, University of Bucharest, Romania;
Associate of the Abdus Salam International Centre for Theoretical Physics, Trieste, Italy;
Research Associate of the Johns Hopkins University, School of Medicine, MR Research Division, Baltimore, MD, USA.
E-mail mutihac@rdslink.ro
Keywords:
Abstract: Biomedical signals are a rich source of information about physiological processes, though typically being mixtures of unknown combinations of sources summing differently at different loci, and often contaminated with artifacts and/or noise. Further, for many data sets even the nature of the sources is an open question. Beyond the plain imaging of morphological structure, the analysis and visualization of biomedical image time series data is a challenge with growing importance for both basic research and clinical application. In this respect, functional magnetic resonance imaging (fMRI), as a non-invasive technique in localizing dynamic brain processes in intact living brain, is by far the most complex and informative approach in neuroscience imaging. Specifically, fMRI is used to track brain function by visualizing changes in chemical composition of brain areas or changes in the flow of fluids that occur over time spans of seconds to minutes. It is based on the differing magnetic susceptibility of the oxygenated hemoglobin (HbO2) and the deoxygenated hemoglobin (HbR), tracking blood-flow-related phenomena accompanying or following neuronal activations.
The classical principle behind detecting activations using fMRI is essentially a voxel-by-voxel test of some statistics against the null hypothesis (e.g., no activation) on a series of images acquired under different conditions. The index of neuronal activity largely used in brain imaging is the blood oxygenation level dependent (BOLD) contrast. The basic assumption is that an increase in neuronal activity within a brain region entails an increase in local blood flow, leading to reduced concentrations of deoxyhemoglobin (HbR) in the blood vessels. While HbO2 is paramagnetic , HbR is diamagnetic , which implies a different in relation to the surrounding tissue. Therefore, relative decreases in HbR concentration attract a reduction in local field inhomogeneity and a slower decay of the MR signal, resulting in higher intensities of spin-spin relaxation time weighted images. The analysis of fMRI brain data is a complex task, since the fMRI signals have relatively varied and unpredictable time courses that represent the summation of signals from hemodynamic changes as a result of neural activities, from subject motion and scanner artifacts, and from physiological cardiac, respiratory and other pulsations. The relative contribution and exact form of each of these components are largely unknown, suggesting exploratory analysis methods based on data structure (data-driven model-free approach) rather than prior hypotheses (hypothesis-driven statistical inference).
A general trend in data analysis consists in finding an adequate representation of multivariate data, which is expected to provide the underlying factors describing their origin and essential structure. Some common linear transformation methods are principal component analysis (PCA), factor analysis (FA), projection pursuit (PP), and, more recently, the fuzzy cluster analysis (FCA) and the independent component analysis (ICA). The latter has emerged as a useful extension of nonlinear PCA and developed in context of blind source separation (BSS) and digital signal processing (DSP). ICA is also related to recent theories of the visual brain, which assume that consecutive processing steps lead to a progressive reduction in the redundancy of visual representation; last but not least, ICA has been invoked in research on sparse and low entropy coding.
The present contribution addresses the highs and lows of various univariate/multivariate hypothesis/data-driven methods of biomedical time series analysis, applied to an fMRI case study of a visuo-motor paradigm. The analysis of large and complex data sets is efficiently performed by two overlapping classes of techniques known as exploratory data analysis (EDA) and data mining. EDA helps to cope with data in a fairly informal way and reveals structure relatively quickly and easy. The emphasis is on flexible probing of data, often before comparing them to any probabilistic model. EDA is used to identify systematic relations between variables when there are no (or not complete) a priori expectations as to the nature of those relations. Data mining is an analytic process designed to explore usually large amounts of data in search of consistent patterns and/or systematic relationships between variables, and then to validate the findings by applying the detected patterns to new subsets of data. An important difference in the focus and purpose between data mining and EDA is that data mining is more oriented towards applications rather than the basic nature of the underlying phenomena. EDA and data mining emphasize flexible searching for clues and evidence in large data sets, whereas confirmatory data analysis (CDA) stresses evaluating the available evidence and assessing the statistical power/significance. These two categories are complementary and mirror the exploratory and confirmatory aspects of scientific investigation. Imaging studies driven by hypotheses derived from cognitive psychology and related disciplines can at best support or refute currently formulated psychological models. Unanticipated time courses of activation of localized brain areas are less likely to be discovered with such analysis methods.
Frequently, fMRI experiments reveal co-activation of spatially disparate brain regions, which cannot be rigorously investigated with univariate techniques, because they examine each voxel individually to determine if a given voxel is deemed task-related by a specified criterion and ignore the relationships between voxels. Contrarily, multivariate techniques separate data into a set of spatial patterns of activity (maps), enabling the analysis of co-activation in spatially divergent areas within a given map. In data-driven analysis, no statistical inference model needs to specify. Multivariate data analysis relies on the covariance paradigm and is free of prior assumptions on activation functions. EDA is capable to detect the functional activity without reference to the experimental protocol and can also reveal new components in the data. Two features of fMRI data are nonstationarity and distributional heterogeneity, which may adversely affect the assumptions of the ICA model. Yet many aspects of fMRI signals are quite well known and could be incorporated into ICA. If the activations do not have a systematic overlap in time and/or space, then the distributions can be considered independent. The assumption that components are spatially independent and add linearly was evaluated and it was concluded that the fMRI signals and some noise are non-Gaussian, though the accuracy of the ICA model may vary in specific regions of the brain.
The experimental data originated from 36 healthy right-handed subjects, equally males and females, performing sessions of single-shot MR EPI scanning at 1.5T magnetic field. The experimental paradigm was a visuo-motor task consisted in rapidly presented color arrows every 1.5s for 150ms, calling upon subjects to press a response button when the prevalent (80%) green arrows pointing right (“Right”) were displayed but not to the infrequent (20%) red arrows pointing left (“Left”). All sessions lasted 4min 50s, each session including 6 periods of fixation of 10s. The acquisition matrix was and the voxel size was mm3 with 0.5 mm gap between slices. 120 volumes/session were acquired at seconds, and the first 6 acquired volumes were discarded as dummies, aiming to reach a steady-state regime. The exploratory analysis (ICA) allowed straightforward analysis of more complex brain imaging experiments in which unpredictable changes in cognitive activation occur in parallel with changes in arousal or autonomic states for which the exact time courses of activation are not known. The findings revealed “auditory” activation due to coil gradient noise during a “non-auditory” task. This can be modeled as a new regressor in the general linear model (GLM) in statistical parametric mapping (SPM) with benefits in model accuracy and physiological interpretation for much more complex paradigms.
Applying exploratory analysis of neuroimaging data augments the ongoing re-evaluation of inferential statistics in imaging neuroscience. Future techniques are likely to combine data- and hypothesis-driven analysis approaches by initially employing powerful data-driven methods to reveal the underlying nature of the signals and noise and, subsequently, testing hypotheses of interest within the previously determined more accurate context, such as multivariate linear models.
|
|
|
|