|
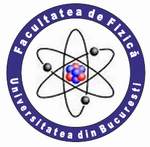 |
UNIVERSITY OF BUCHAREST FACULTY OF PHYSICS Guest 2025-07-05 8:02 |
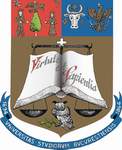 |
|
|
Conference: Bucharest University Faculty of Physics 2009 Meeting
Section: Biophysics; Medical Physics
Title: Fractal complexity of the human brain cortex
Authors: Radu Mutihac
Affiliation: Faculty of Physics, University of Bucharest
E-mail mutihac@astralnet.ro
Keywords: Multiresolution analysis, fractals, wavelets, human brain
Abstract: In nature, various wonderful complicated shapes such as coastlines, lightnings, clouds, and plants display some similar characteristics. To measure such complicated shapes, Benoit Mandelbrot introduced a new concept in mathematics called “fractal” in 1975. Brain function depends on adaptive self-organization of large-scale neural assemblies. As such, the human brains may be analyzed as if the human cerebral cortex is self similar in a statistical sense, a property which is usually referred to as being a fractal. The analysis hereafter includes all spatial scales from the brain size to the ultimate image resolution.
Fractals define a class of objects with the characteristic property of self-similarity (or self-affinity), meaning that the statistics describing the structure in time or space of a fractal process remain the same as the process is measured over a range of different scales (or scale invariant). Fractals are complex, patterned, statistically self-similar or self-affine, scaling or scale-invariant structures with non-integer dimensions, generated by simple iterative rules widespread in real and synthetic systems.
Brain analysis methods, which reveal statistical regularities in data associated with brain function, can loosely be dichotomized in: (i) hypothesis-driven (confirmatory) and (ii) data-driven (exploratory) analysis. In statistical inference, the expected changes in brain activity are specified as regressors within a multiple linear regression framework and the estimated regression coefficients are tested against a null hypothesis. The voxelwise test statistics form summary maps that are representations of the spatial distribution of functional activity induced by some experimental paradigms. Contrarily, exploratory analysis makes no reference to prior knowledge of data structure and provides models whose characteristics are solely determined by statistical properties of data.
Multiscale analysis falls somewhat in between since the key features of signals are identified and analyzed with resolutions matched to their scales and subsequently assessed for statistical significance. The approach is optimal in that of detecting transient events and adapting to local or nonstationary features in data within decomposition scales.
Wavelets are fractal in nature and so an appropriate basis for analysis of fractal data. As such, wavelet methods are particularly adequate in brain imaging data analysis due to broadly fractal properties exhibited by the brain in space and time. Hence wavelets constitute natural rather than just another basis for functional neuroimaging data processing revealing activity patterns based on multiresolution analysis at suitable scales.
|
|
|
|