|
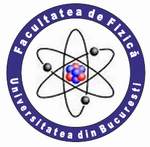 |
UNIVERSITY OF BUCHAREST FACULTY OF PHYSICS Guest 2025-04-04 7:33 |
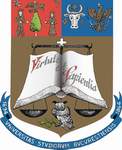 |
|
|
Conference: Bucharest University Faculty of Physics 2015 Meeting
Section: Biophysics; Medical Physics
Title: Functional Magnetic Resonance Imaging
– Inferential Statistics versus Exploratory Data Analysis –
Authors: Simona SPÎNU (1), Radu MUTIHAC (1)
Affiliation: (1) University of Bucharest, Faculty of Physics, Magurele, Romania
E-mail simonaspinu91@yahoo.com
Keywords: Magnetic resonance imaging (MRI), functional magnetic resonance imaging (fMRI), statistical parametric mapping (SPM), independent component analysis (ICA), fuzzy cluster analysis (FCA), exploratory data analysis (EDA), confirmatory data analysis(CDA), gen
Abstract: Imaging Neuroscience is aiming to reveal functional changes in brain activity and structural changes in neuroanatomy. Magnetic resonance imaging (MRI) is a noninvasive imaging modality used to visualize virtually all internal human body structures including brain too. More recently, functional MRI (fMRI) has emerged as a functional neuroimaging procedure that measures brain activity by detecting associated changes in blood flow on the basis of blood oxygenation level-dependent (BOLD) contrast. An fMRI investigation comes out with large and complex data sets that need to be statistically analyzed and interpreted by computers running specific programs like statistical parametric mapping (SPM), independent component analysis (ICA) or fuzzy cluster analysis (FCA).
The present research work high lights the advances in functional MR neuroimaging by critically comparing the confirmatory (CDA) and exploratory data analysis (EDA) of real-life fMRI time series. Typical exploratory multivariate methods like ICA and FCA were applied to functional neuroimaging data and comparatively discussed versus univariate inferential statistical techniques like the generalized linear model (GLM) within the framework of SPM.
Spatiotemporal characteristics of brain activity are frequently unknown and variable, which preclude their evaluation by confirmatory methods only. Revealing unanticipated or missed patterns of activation, EDA makes no reference to prior knowledge and provides models solely based on the statistical properties of data. Likewise, it allows to improve or even to change the original working hypotheses. Whereas EDA is searching for evidence in data, CDA is evaluating the available evidence, which render them complementary rather than competitive.
In imaging Neuroscience, the dynamic interplay between hypothesis generation and hypothesis testing, a Hegelian synthesis of EDA and CDA, appears optimal in dealing with the increasingly complex experiments, or the emerging broad range of theoretical and clinical studies.
|
|
|
|