|
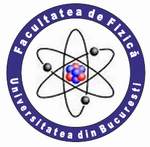 |
UNIVERSITY OF BUCHAREST FACULTY OF PHYSICS Guest 2025-07-09 17:20 |
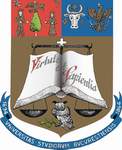 |
|
|
Conference: Bucharest University Faculty of Physics 2022 Meeting
Section: Theoretical Physics and Applied Mathematics
Title: Machine Learning Applications in Anomaly Detection for BSM Searches and Hardware Data Acquisition
Triggering
Authors: loan-Mihail DINU (1,2)
*
Affiliation: 1) Facultatea de Fizica, Universitatea Bucuresti
2) École Doctoral des Sciences Fondamentales, Université Clermont Auvergne
E-mail loan-Mihail.Dinu@cern.ch
Keywords: machine learning, bsm, anomaly detection, trigger
Abstract: Machine Learning (ML) methods have seen increasing adoption rates within High Energy Physics (HEP)
research. and this work attemots to continue those efforts in two critical areas of apolicability: exoerimental
physics analysis and particle data acquisition triggering for particle detectors.
Although most Beyond Standard Model (BSM) searches target specific theory models, there has always
been a keen interest in developing model-independent methods in the HEP community. ML-based anomaly
detection stands among the latest up-and-coming avenues tor creating model-agnostic BSM searches. This
part of the research focuses on designing anomalous event taggers based on autoencoder models. Alongside
the sana aschminauon power. a nan ororiv is paced on bow sana-mo an
independence. To this end, the autoencoder is used in conjunction with a Normalizing Flow model tasked with
latent space density estimation. Both event reconstruction error and latent representation likelihood are
combined to mitigate the bias of the resulting event anomaly score. Overall this method is showing promising
anomaly detection pertormance without losing much in terms or generalization power. Un the multiet LHC
Olympics data, it can consistently identify BSM signals, even in the challenging scenarios posed by the Black
Box datasets, where the signal content Is unknown.
The hardware trigger application focuses on Micromegas-tvoe detectors used for muon detection from
high-energy p - p collisions. The data acquisition trigger should identify tracks originating from the
interaction point while rejecting both collision and non-collision backgrounds. We trained a convolutional
neural network using simulated muons to identify muon tracks and determine if they originate from the
supposed interaction point. This part or the work aims to study the teaslbllity or using machine learning
models on FPGA for trigger algorithm implementation. Our results show that this approach is suitable and can
provide a very good precision.
References:
LHCP Conference proceedings: 10.22323/1.397.0340
LHC Olympics Paper: https://doi.org/10.1088%2F1361-6633%2Fac36b9
|
|
|
|