|
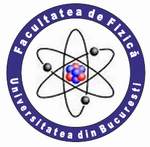 |
UNIVERSITY OF BUCHAREST FACULTY OF PHYSICS Guest 2025-06-15 15:20 |
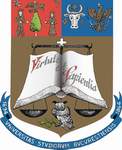 |
|
|
Conference: Bucharest University Faculty of Physics 2023 Meeting
Section: Theoretical Physics and Applied Mathematics
Title: Mapping confinement potentials and charge densities of interacting quantum systems using conditional generative adversarial networks
Authors: Calin Andrei PANTIS-SIMUT(1,2,3), Amanda Teodora PREDA(1,2,3), Lucian ION(1), Andrei MANOLESCU(4) George Alexandru NEMNES(1,2,3)
*
Affiliation: 1) University of Bucharest, Faculty of Physics, 077125 Magurele-Ilfov, Romania
2) Research Institute of the University of Bucharest (ICUB), Sos. Panduri 90, Bucharest Romania.
3) Horia Hulubei National Institute of Physics and Nuclear Engineering (IFIN-HH), Str. Reactorului no.30, P.O.BOX MG-6, Magurele, Romania
4) Department of Engineering, Reykjavik University, Menntavegur 1, Reykjavik, IS-102, Iceland
E-mail caly_pantis98@yahoo.com
Keywords: Many-Body, Exact Diagonalization, Machine Learning, GANs
Abstract: An efficient and accurate description of the many-body states lays the foundation for nanoelectronic devices and applications in quantum information. These require a description beyond mean-field approximation, using methods such as exact diagonalization. The procedure is computationally demanding and for a large number of electrons in the system, it may become impractical. New techniques, based on machine learning, may provide a powerful improvement in the computational time reduction, while providing good accuracy.
In our study, a general-purpose image-to-image translation method, namely pix2pix[1] is engaged. This is an Ansatz free technique, based on conditional generative adversarial network (cGAN), used to predict the ground state densities of the interacting system from randomly generated confinement potentials. Additional mappings were performed, from confinement potential to non-interacting densities and from non-interacting densities to the interacting ones. The inverse problem, namely mapping the interacting charge density to a confinement potential, can be solved by the pix2pix method. Still, not every given charge density is a ground state one or has a corresponding potential, but this inverse problem can provide near-optimal solutions. The technique optimization was performed by varying Generator, Discriminator architectures, and other parameters.
References:
P. Isola, J.-Y. Zhu, T. Zhou, A. A. Efros, Image-to-image translation with conditional adversarial networks (2016). doi:10.48550/ARXIV.1611.07004.
Acknowledgement: This work was supported by a grant of the Romanian Ministry of Research, Innovation and Digitalization, CNCS - UEFISCDI, project number PN-III-P4-ID-PCE-2020-1142, within PNCDI III.
|
|
|
|