|
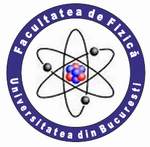 |
UNIVERSITY OF BUCHAREST FACULTY OF PHYSICS Guest 2025-04-26 10:25 |
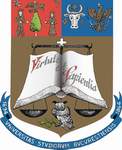 |
|
|
Conference: Bucharest University Faculty of Physics 2024 Meeting
Section: Biophysics; Medical Physics
Title: AI methods for the analysis of Stereoelectroencephalographic recordings
Authors: Roxana-Andreea BADEA (1,2), Constantin Augustin Dan PISTOL (1), Andrei BARBORICĂ (1)
Affiliation: 1) University of Bucharest, Faculty of Physics, Department of Electricity, Solid-State Physics and Biophysics,Atomistilor Street 405, 077125 Măgurele, Ilfov, Romania
2) Horia Hulubei National Institute for R&D in Physics and Nuclear Engineering, Str. Reactorului 30, RO-077125, P.O.B. MG-6, Măgurele-Bucharest, Romania
E-mail roxana-andreea.badea1@s.unibuc.ro
Keywords: EEG, SEEG, IED spikes, Python, AI, CNN, Machine Learning
Abstract: This paper explores the application of artificial intelligence methods to the analysis of stereoelectrographic recordings, with the primary aim of improving the detection of interictal spikes (IED) by increasing the values of sensitivity, precision, and accuracy. Time-frequency maps were generated for multiple recording channels, and a convolutional neural network (CNN) was employed. This CNN underwent a fine-tuning process to adapt it to the specific characteristics of the dataset. The algorithm was run on the dataset from the N. Roehri database, where the ground truth consisted of spike markers that indicate interictal spikes. These markers were manually annotated by the authors of the dataset using AnyWave. This dataset contains 960 channels, with each channel having an average of 25 spikes. Initially, the pre-trained model applied to one channel from the N. Roehri dataset achieved approximately 61% sensitivity, 42% accuracy, and 88% precision. After fine-tuning, the model was tested on new channels from the dataset to evaluate its performance. The results of the fine-tuned CNN were then compared with the ground truth on several channels, as well as with the initial results obtained from the pre-trained model on the same channels prior to fine-tuning. Additionally, the study underscores the efficacy and applicability of the proposed method in the field of neurological signal analysis. The results indicate that the adapted CNN can serve as a powerful tool for the detailed analysis of stereoelectrographic data, providing insights that are crucial for both clinical and research applications in neurology.
References:
1. Roehri N., Pizzo F., Bartolomei F., Wendling F., Bénar C. G., What are the assets and weaknesses of HFO detectors? A benchmark framework based on realistic simulations, PLoS One, 12, 4, 2017, doi: 10.1371/journal.pone.0174702.
2. Pizzo F. et al., The Ictal Signature of Thalamus and Basal Ganglia in Focal Epilepsy: A SEEG Study, Neurology, 96, 2, e280–e293, 2021, doi: 10.1212/WNL.0000000000011003.
3. Quon R. J. et al., AiED: Artificial intelligence for the detection of intracranial interictal epileptiform discharges, Clinical Neurophysiology, 133, 1–8, 2022, doi: 10.1016/j.clinph.2021.09.018.
|
|
|
|